Mapping of Artificial Intelligence and Robotics Technologies Applied to Offshore Wind Energy
DOI:
https://doi.org/10.24883/eagleSustainable.v15i.474Palavras-chave:
Artificial Intelligence, Robotics, Offshore Wind Farm, Systematic literature reviewResumo
Objective: this paper aims to map the main artificial intelligence and robotics technologies that are being applied in offshore wind farms around the world, as well as highlight the possible classification of these technologies in Brazil.
Methodology/approach: the methodology of the work consists of carrying out a bibliometric study based on a Scopus database where a series of quantitative and qualitative analyses were made and, finally, the main papers were grouped into 8 central clusters found.
Originality/Relevance: The relevance of the work consists of presenting to researchers the main fields that have been studied in the applications of AI and robotics in the context of offshore wind farms and, therefore, allows new research to occur in these fields found from the clusters. In addition, the work summarizes in which stages throughout the development of offshore projects each of the clusters can be applied, thus allowing a significant advance for possible projects to be carried out in Brazil in the future.
Main conclusions: as a result of the research, eight main clusters of research carried out in the field were identified, as well as their possible classification in the Brazilian scenario in the future.
Theoretical/methodological contributions: the scientific contributions that the paper presents to researchers are diverse, among which we can list: the mapping of the main journals that have publications on the theme of AI and robotics applications in the field of offshore wind energy, the main trends in AI and robotics technologies applied to offshore wind energy around the world and, finally, the mapping of the most relevant paper on AI and robotics applications in the context of offshore wind energy, as well as their evidence in the Brazilian context.
Downloads
Referências
Ahuir-Torres, J. I., Souto, R. M., Lozano, R., de la Fuente, I. M., & González-García, Y. (2019). Benchmarking parameters for remote electrochemical corrosion detection and monitoring of offshore wind turbine structures. Wind Energy, 22(6), 857-876. https://doi.org/10.1002/we.2362 DOI: https://doi.org/10.1002/we.2324
Antoniadou, I., Dervilis, N., Papatheou, E., Maguire, A. E., & Worden, K. (2015). Aspects of structural health and condition monitoring of offshore wind turbines. Philosophical Transactions of the Royal Society A: Mathematical, Physical and Engineering Sciences. https://doi.org/10.1098/rsta.2014.0075 DOI: https://doi.org/10.1098/rsta.2014.0075
Ali, S. W., Wang, L., Wu, X., Rehman, M. H., & Rashid, M. (2021). Offshore wind farm-grid integration: A review on infrastructure, challenges, and grid solutions. IEEE Access, 9, 102811-102827. https://doi.org/10.1109/ACCESS.2021.3099302 DOI: https://doi.org/10.1109/ACCESS.2021.3098705
Archer, C. L., Delle Monache, L., Rife, D. L., & Lundquist, J. K. (2017). The challenge of integrating offshore wind power in the US electric grid. Part I: Wind forecast error. Renewable Energy, 103, 346-360. https://doi.org/10.1016/j.renene.2016.11.047 DOI: https://doi.org/10.1016/j.renene.2016.11.047
Balluff, S., Bendfeld, J., & Krauter, S. (2015). Short term wind and energy prediction for offshore wind farms using neural networks. In 2015 International Conference on Renewable Energy Research and Applications (ICRERA) (pp. 379–382). IEEE. https://doi.org/10.1109/ICRERA.2015.7418472 DOI: https://doi.org/10.1109/ICRERA.2015.7418440
Chan, H.-C., Chen, C.-S., & Hsieh, C.-Y. (2013). Preliminary plan of underwater environmental monitoring in the offshore wind farm in the western sea of Taiwan. In 2013 MTS/IEEE OCEANS-Bergen (pp. 1-4). IEEE. https://doi.org/10.1109/OCEANS-Bergen.2013.6608132 DOI: https://doi.org/10.1109/OCEANS-Bergen.2013.6608093
Campos, F. R. (2019). A robótica para uso educacional. BOD GmbH DE.
Chatterjee, J., & Dethlefs, N. (2021). Scientometric review of artificial intelligence for operations & maintenance of wind turbines: The past, present and future. Renewable and Sustainable Energy Reviews,v.373. https://doi.org/10.1098/rsta.2014.0075 DOI: https://doi.org/10.1016/j.rser.2021.111051
Ding, X., Li, Y., Zhang, W., & Zhao, H. (2024). Artificial intelligence based abnormal detection system and method for wind power equipment. International Journal of Thermofluids, 21, 100569. https://doi.org/10.1016/j.ijft.2023.100569 DOI: https://doi.org/10.1016/j.ijft.2024.100569
Ditria, E. M., Lopez-Marcano, S., Sievers, M., Jinks, E. L., Brown, C. J., & Connolly, R. M. (2022). Artificial intelligence and automated monitoring for assisting conservation of marine ecosystems: A perspective. Frontiers in Marine Science, 9, 918104. https://doi.org/10.3389/fmars.2022.918104 DOI: https://doi.org/10.3389/fmars.2022.918104
International Renewable Energy Agency. (2023). Enabling frameworks for offshore wind scale up: Innovations in permitting. [S. l.]. Available at: https://www.irena.org/Publications/2023/Sep/Enabling-frameworks-for-offshore-wind-scale-up. Accessed on: August 10, 2024.
Fischetti, M., & Fraccaro, M. (2019). Machine learning meets mathematical optimization to predict the optimal production of offshore wind parks. Computers & Operations Research, 106, 289-297. https://doi.org/10.1016/j.cor.2018.11.006 DOI: https://doi.org/10.1016/j.cor.2018.04.006
Gehring, C., Schreiber, M., & Fuchs, H. (2021). ANYmal in the field: Solving industrial inspection of an offshore HVDC platform with a quadrupedal robot. In Field and Service Robotics: Results of the 12th International Conference (pp. 247-260). Springer Singapore. https://doi.org/10.1007/978-981-16-0247-3_19 DOI: https://doi.org/10.1007/978-981-15-9460-1_18
Gonzalez-Longatt, F. M., Wall, P., Regulski, P., & Terzija, V. (2011). Optimal electric network design for a large offshore wind farm based on a modified genetic algorithm approach. IEEE Systems Journal, 5(2), 268–276. https://doi.org/10.1109/JSYST.2011.2163027 DOI: https://doi.org/10.1109/JSYST.2011.2163027
Gu, Y., Zhang, L., Li, Y., & Liu, X. (2024). Vessel intrusion interception utilizing unmanned surface vehicles for offshore wind farm asset protection. Ocean Engineering, 299, 117395. https://doi.org/10.1016/j.oceaneng.2024.117395 DOI: https://doi.org/10.1016/j.oceaneng.2024.117395
Hou, P., Liu, X., & Zhang, Z. (2019). A review of offshore wind farm layout optimization and electrical system design methods. Journal of Modern Power Systems and Clean Energy, 7(5), 975-986. https://doi.org/10.35833/MPCE.2018.000718
Hou, P., et al. (2019). A review of offshore wind farm layout optimization and electrical system design methods. Journal of Modern Power Systems and Clean Energy, 7(5), 975–986. https://10.1007/s40565-019-0550-5 DOI: https://doi.org/10.1007/s40565-019-0550-5
Ingram, E. C., & Butler, L. (2024). Artificial intelligence and species distribution ensemble models inform resource interactions with offshore wind development. bioRxiv. https://10.1101/2024.06.10.598232 DOI: https://doi.org/10.1101/2024.06.10.598232
Khalid, O., Ali, M., Qureshi, A., & Saeed, K. (2022). Applications of robotics in floating offshore wind farm operations and maintenance: Literature review and trends. Wind Energy, 25(11), 1880-1899. https://doi.org/10.1002/we.2663 DOI: https://doi.org/10.1002/we.2773
Kokash, N. (2005). An introduction to heuristic algorithms. Department of Informatics and Telecommunications, 1-8.
Kou, L., Zhang, W., & Li, H. (2022). Review on monitoring, operation and maintenance of smart offshore wind farms. Sensors, 22(8), 2822. https://doi.org/10.3390/s22082822 DOI: https://doi.org/10.3390/s22082822
Lawan, S. M., Ibrahim, A., & Alkali, A. (2014). Different models of wind speed prediction; a comprehensive review. International Journal of Scientific & Engineering Research, 5(1), 1760-1768.
Leonard, J. J., & Bahr, A. (2016). Autonomous underwater vehicle navigation. In Springer handbook of ocean engineering (pp. 341-358). Springer. https://doi.org/10.1007/978-3-319-24115-4_21 DOI: https://doi.org/10.1007/978-3-319-16649-0_14
Lozano-Minguez, E., Kolios, A. J., & Brennan, F. P. (2011). Multi-criteria assessment of offshore wind turbine support structures. Renewable Energy, 36(11), 2831-2837. https://doi.org/10.1016/j.renene.2011.04.010 DOI: https://doi.org/10.1016/j.renene.2011.04.020
Mitchell, D., Blanche, J., Harper, S., Lim, T., Gupta, R., Zaki, O., & Flynn, D. (2022). A review: Challenges and opportunities for artificial intelligence and robotics in the offshore wind sector. Energy and AI, 8, 100146. https://doi.org/10.1016/j.egyai.2022.100146 DOI: https://doi.org/10.1016/j.egyai.2022.100146
Neshat, M., Zhang, L., & Moosavi, R. (2021). A deep learning-based evolutionary model for short-term wind speed forecasting: A case study of the Lillgrund offshore wind farm. Energy Conversion and Management, 236, 114002. https://doi.org/10.1016/j.enconman.2021.114002 DOI: https://doi.org/10.1016/j.enconman.2021.114002
Niemi, J., & Tanttu, J. T. (2020). Deep learning–based automatic bird identification system for offshore wind farms. Wind Energy, 23(6), 1394-1407. https://doi.org/10.1002/we.2578 DOI: https://doi.org/10.1002/we.2492
Noronha, M., Pimentel, R. F., & Pinho, M. R. (2021). The role of the innovation ecosystem and the structuring of a regulatory framework for the offshore wind energy market in Brazil. International Journal of Business and Marketing, 6(2), 32-51. https://doi.org/10.1002/bm.10001
Papatheou, E., Dervilis, N., Maguire, A. E., Antoniadou, I., & Worden, K. (2015). A performance monitoring approach for the novel Lillgrund offshore wind farm. IEEE Transactions on Industrial Electronics, 62(10), 6636–6644. https://doi.org/10.1109/TIE.2015.2442212 DOI: https://doi.org/10.1109/TIE.2015.2442212
Parsons, H. McIlvaine, & Kearsley, G. P. (1982). Robotics and human factors: Current status and future prospects. Human Factors, 24(5), 535-552. https://doi.org/10.1177/1937586717747384 DOI: https://doi.org/10.1177/001872088202400504
Pati, D., & Lorusso, L. N. (2018). How to write a systematic review of the literature. HERD: Health Environments Research & Design Journal, 11(1), 15-30. DOI: https://doi.org/10.1177/1937586717747384
Paula, M., dos Santos, A. R., & da Silva, J. (2023). Predicting energy generation in large wind farms: A data-driven study with open data and machine learning. Inventions, 8(5), 126. https://doi.org/10.3390/inventions8050126 DOI: https://doi.org/10.3390/inventions8050126
Paula, M., dos Santos, A. R., & Guedes Soares, C. (2020). Predicting long-term wind speed in wind farms of northeast Brazil: A comparative analysis through machine learning models. IEEE Latin America Transactions, 18(11), 2011-2018. https://doi.org/10.1109/TLA.2020.9348816 DOI: https://doi.org/10.1109/TLA.2020.9398643
Pencelli, M., Mendez, C., & Gálvez, E. (2024). Testing the robustness of quadruped robots for unmanned inspection activities in the energy industry. In International Petroleum Technology Conference (p. D021S083R008). IPTC. DOI: https://doi.org/10.2523/IPTC-24373-MS
Pillai, A. C., Venkatesan, S., & Kumar, S. (2016). Optimisation of offshore wind farms using a genetic algorithm. DOI: https://doi.org/10.17736/ijope.2016.mmr16
International Journal of Offshore and Polar Engineering, 26(03), 225-234. https://doi.org/10.1773/ijope.v26i3.225
Rinaldi, G., Thies, P. R., & Johanning, L. (2021). Current status and future trends in the operation and maintenance of offshore wind turbines: A review. Energies, 14(9), 2484. https://doi.org/10.3390/en14092484 DOI: https://doi.org/10.3390/en14092484
Ren, Z., Verma, A. S., Li, Y., Teuwen, J. J., & Jiang, Z. (2021). Offshore wind turbine operations and maintenance: A state-of-the-art review. Renewable and Sustainable Energy Reviews, v.144. https://doi.org/10.1016/j.rser.2021.110886 DOI: https://doi.org/10.1016/j.rser.2021.110886
Rother, E. T. (2007). Systematic literature review X narrative review. Acta paulista de enfermagem, 20, v-vi. DOI: https://doi.org/10.1590/S0103-21002007000200001
Sacie, M., Sousa, D. F., & Oliveira, F. (2022). Use of state-of-art machine learning technologies for forecasting offshore wind speed, wave and misalignment to improve wind turbine performance. Journal of Marine Science and Engineering, 10(7), 938. https://doi.org/10.3390/jmse10070938 DOI: https://doi.org/10.3390/jmse10070938
Shafiee, M., Zhou, Z., Mei, L., Dinmohammadi, F., Karama, J., & Flynn, D. (2021). Unmanned aerial drones for inspection of offshore wind turbines: A mission-critical failure analysis. Robotics, v.10. https://doi.org/10.3390/robotics10010026 DOI: https://doi.org/10.3390/robotics10010026
Salkanović, E., Enevoldsen, P., & Xydis, G. (2020). Applying AI-Based solutions to avoid bird collisions at wind parks. In Complementary Resources for Tomorrow: Proceedings of Energy & Resources for Tomorrow 2019 (pp. 111-124). Springer International Publishing. https://doi.org/10.1007/978-3-030-59254-8_10 DOI: https://doi.org/10.1007/978-3-030-38804-1_7
Sattar, T. P., Leon Rodriguez, H., & Bridge, B. (2009). Climbing ring robot for inspection of offshore wind turbines. Industrial Robot: An International Journal, 36(4), 326–330. https://doi.org/10.1108/01439910910957075
Salvador, S., Gimeno, L., & Larruga, F. J. S. (2018). The influence of regulatory framework on environmental impact assessment in the development of offshore wind farms in Spain: Issues, challenges and solutions. Ocean & Coastal Management, 161, 165-176. https://doi.org/10.1016/j.ocecoaman.2018.04.016 DOI: https://doi.org/10.1016/j.ocecoaman.2018.05.010
Santos, F. P., Teixeira, A. P., & Guedes Soares, C. (2015). An age-based preventive maintenance for offshore wind turbines. In Safety and Reliability: Methodology and Applications (pp. 1147-1155).
Sattar, T. P., Leon Rodriguez, H., & Bridge, B. (2009). Climbing ring robot for inspection of offshore wind turbines. Industrial Robot: An International Journal, 36(4), 326-330. https://doi.org/10.1108/01439910910965289 DOI: https://doi.org/10.1108/01439910910957075
Schneider, J., Klüner, A., & Zielinski, O. (2023). Towards digital twins of the oceans: The potential of machine learning for monitoring the impacts of offshore wind farms on marine environments. Sensors, 23(10), 4581. https://doi.org/10.3390/s23104581 DOI: https://doi.org/10.3390/s23104581
Silva, A. J. S. F. da, de Sousa, A. J. S., & Reis, M. (2010). Técnicas de inteligência artificial e controlo adaptativo aplicadas à gestão de parques eólicos.
Soman, S. S., Dhingra, S., & Shinde, A. (2010). A review of wind power and wind speed forecasting methods with different time horizons. In North American Power Symposium 2010 (pp. 1-8). IEEE. DOI: https://doi.org/10.1109/NAPS.2010.5619586
Song, D., Wang, L., & Chen, Y. (2024). Review on the application of artificial intelligence methods in the control and design of offshore wind power systems. Journal of Marine Science and Engineering, 12(3), 424. https://doi.org/10.3390/jmse12030424 DOI: https://doi.org/10.3390/jmse12030424
Vijayalakshmi, S., V, A., & Rahman, M. (2023). The role of artificial intelligence in renewable energy. In AI-Powered IoT in the Energy Industry: Digital Technology and Sustainable Energy Systems (pp. 253-269). Cham: Springer International Publishing. https://doi.org/10.1007/978-3-030-90556-3_15 DOI: https://doi.org/10.1007/978-3-031-15044-9_12
Vinuesa, R., Aziz, W., & Hossain, M. (2020). The role of artificial intelligence in achieving the Sustainable Development Goals. Nature Communications, 11(1), 1-10. https://doi.org/10.1038/s41467-020-17528-8 DOI: https://doi.org/10.1038/s41467-019-14108-y
Winston, P. H. (1984). Artificial intelligence. Addison-Wesley Longman Publishing Co., Inc.
Yang, P., Li, M., & Zheng, J. (2022). Design and control of a crawler-type wall-climbing robot system for measuring the paint film thickness of offshore wind turbine tower. Journal of Intelligent & Robotic Systems, 106(2), 50. https://doi.org/10.1007/s10846-021-01482-3 DOI: https://doi.org/10.1007/s10846-022-01750-w
Yeter, B., Garbatov, Y., & Guedes Soares, C. (2022). Life-extension classification of offshore wind assets using unsupervised machine learning. *Reliability Engineering & System DOI: https://doi.org/10.1016/j.ress.2021.108229
Yu, M., Zhang, Z., Li, X., Yu, J., Gao, J., Liu, Z., You, B., Zheng, X., & Yu, R. (2020). Superposition graph neural network for offshore wind power prediction. Future Generation Computer Systems, 113, 68–77. https://doi.org/10.1016/j.future.2020.06.024 DOI: https://doi.org/10.1016/j.future.2020.06.024
Zhou, F., Tu, X., & Wang, Q. (2022). Research on offshore wind power system based on Internet of Things technology. International Journal of Low-Carbon Technologies, 17, 645-650. https://doi.org/10.1093/ijlct/ctab027 DOI: https://doi.org/10.1093/ijlct/ctac049
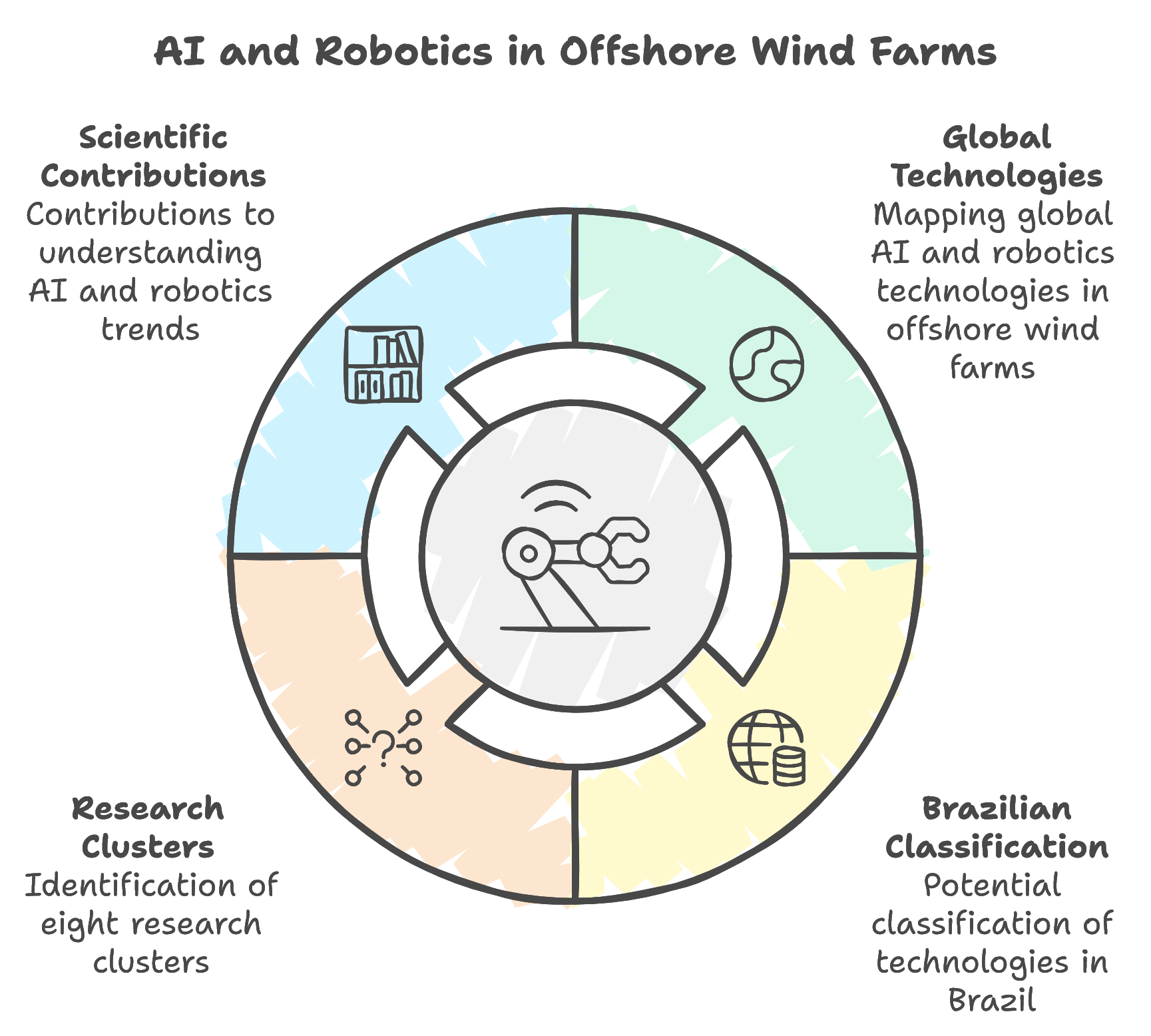
Downloads
Publicado
Como Citar
Edição
Seção
Licença
Copyright (c) 2024 Revista Inteligência Competitiva

Este trabalho está licenciado sob uma licença Creative Commons Attribution 4.0 International License.
O(s) autor(es) autoriza(m) a publicação do texto na da revista;
O(s) autor(es) garantem que a contribuição é original e inédita e que não está em processo de avaliação em outra(s) revista(s);
A revista não se responsabiliza pelas opiniões, idéias e conceitos emitidos nos textos, por serem de inteira responsabilidade de seu(s) autor(es);
É reservado aos editores o direito de proceder a ajustes textuais e de adequação às normas da publicação.
Autores mantém os direitos autorais e concedem à revista o direito de primeira publicação, com o trabalho simultaneamente licenciado sob a Creative Commons Atribuição-NãoComercial 4.0 Internacional.que permite o compartilhamento do trabalho com reconhecimento da autoria e publicação inicial nesta revista.
Esta licença permite que outros remixem, adaptem e criem a partir do seu trabalho para fins não comerciais, e embora os novos trabalhos tenham de lhe atribuir o devido crédito e não possam ser usados para fins comerciais, os usuários não têm de licenciar esses trabalhos derivados sob os mesmos termos.
Autores têm autorização para assumir contratos adicionais separadamente, para distribuição não-exclusiva da versão do trabalho publicada nesta revista (ex.: publicar em repositório institucional ou como capítulo de livro), com reconhecimento de autoria e publicação inicial nesta revista.
Autores têm permissão e são estimulados a publicar e distribuir seu trabalho online (ex.: em repositórios institucionais ou na sua página pessoal) a qualquer ponto antes ou durante o processo editorial, já que isso pode gerar alterações produtivas, bem como aumentar o impacto e a citação do trabalho publicado (Veja O Efeito do Acesso Livre) emhttp://opcit.eprints.org/oacitation-biblio.html